John B. Hey patented the first of several systems that have capitalized on the idea of automating video recommendation. Tracking down the story proves to be quite an adventure.
By Kathleen Maher
Adobe has won a 2013 Technology and Engineering Emmy Award for its role in technology for recommendations for video discovery. Really? There would have been several companies on my list for the “inventors” of recommendation technology, and none of them would have been Adobe. And, as it happens with these things, getting to the bottom of this innocuous announcement was a pretty interesting trip down the information rat hole.
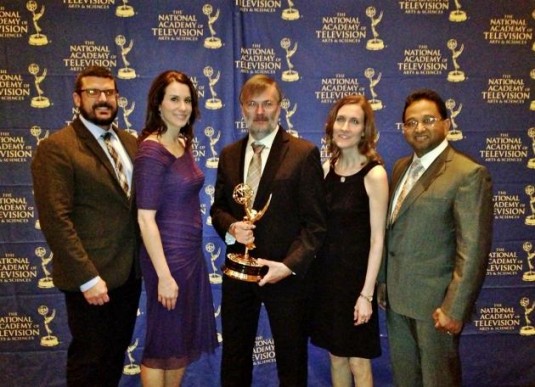
The award recognized John B. Hey for coming up with the idea of a recommendation system and deploying it. Hey originally developed his recommendation system for kiosks to be placed in video stores. He submitted his patent for the technology in 1987. Customers could get recommendations for video rentals by feeding information about their movie likes and dislikes into the kiosks. Hey’s company, LikeMinds, eventually merged with Andromedia, which was acquired by Macromedia in 1999, and of course, Macromedia was acquired by Adobe in 2005 in what turned out to be an astoundingly great idea on the part of Adobe’s management.
Hey continued to expand his work in recommendations including the development of a system for computer-based marketing. That technology, patented in 2001, attempted to predict a consumer’s interest in products including movies, books, commercial products, Web pages, television programs, articles, etc., based on the user’s behavior and that person’s similarities to other users. The system constructs archetypes and groups people into like-minded groups. The approach attempts to then predict what people might want to buy, see, or do even when there are no known preferences for that person.
Researchers at the University of Minnesota developed their own recommendation approach for the Usenet News Groups in response to the rising tide of information being generated by the Internet. They created a system of affinity groups, a process that came to be called “collective filtering.” A member would specify other members they trusted to receive information from and let them choose content they liked. The system included ratings to enable worthy content to float to the top. The group called itself the GroupLens Project.
Meanwhile, back at MIT, engineers at the Media Lab were working on the problem of finding music. The group, led by the Media Lab’s Pattie Maes, also included people from the Harvard Business School. They originally built a recommendation system using collaborative filtering and then developed HOMR (Helpful Online Music Recommendation Service) and eventually created the Firefly system. Firefly existed primarily as a community where people could find and share recommendations, and it expanded to recommendations for movies, websites, and web communities. Barnesandnoble.com, ZDnet, launch.com, and MyYahoo used the system. Interestingly, and not all that relevant, the group become concerned about privacy issues because they were gathering huge amounts of information about people. They worked with the US government to define privacy protection in an effort that obviously didn’t work in all sectors. They also contributed to standards defining privacy practices and helped develop P3P (Platform for Privacy Preferences). All that led to their acquisition by Microsoft, where they helped develop Microsoft Passport.
So where we are today is with systems that use collaborative filters, which are based on people’s past behavior, or content-based filtering, which looks at the content based on pre-defined characteristics and tries to determine similarities. And most often systems use a variety of approaches including Hey’s system using archetypes. Pandora, started in 1999–2000, grew out of the Human Genome Project, which is an example of the content-based approach. The Netflix system is primarily collaborative filtering as it puts us all to work making choices about the content we like and don’t like. Netflix famously refined its algorithms through the Netflix Prize, a contest that promised a million dollars to the group who could get more accurate hits than Netflix’s house system in use from 2006 to 2009. In 2009, BellKor’s Pragmatic Chaos Team won the prize with a system that used as many as 107 different algorithms blended into a single system.
I know, rat hole, right? Blame Mr. John B. Hey.
What do we think?
It was remarkably hard to find information on where Adobe’s technology came from. If the company’s press release had not credited Hey, I would not have found it. I eventually found some patent information, which led to an article by marketers 1-to-1 Media. I had thought Firefly was the original recommendation technology, but Hey’s work was almost a decade earlier. There is so much information on the Internet, and at the same time, there are such giant gaps. So, see this as a modest attempt to fill in one of those gaps.