Benchmarking and assessing a Turing-class GPU a much different process.
At the introduction of its paradigm-shifting Turing generation GPU, Nvidia released three initial versions of Quadro RTX products, the RTX 8000, RTX 6000, and RTX 5000. All are expensive, pricy enough to fit into JPR’s Ultra-high End professional/workstation GPU class, defined as anything over $1,500 street price (and in fact, the RTX 5000 sells at around $2,500 with the 6000 and 8000 up from there). Yes, all those SKUs will sell—and you might be surprised how many—but they serve more as technology-proving marketing showcases for Turing’s potential, especially in the realm of ray tracing and AI acceleration (much more on those features ahead). We’ve been waiting for the most accessible tier, the Quadro 4000-class series typically selling under $1,000—to be refreshed with Turing to get a feel for what Turing can do for a far more significant chunk of the workstation. That time is now, as Nvidia has brought the Quadro RTX 4000 to market.
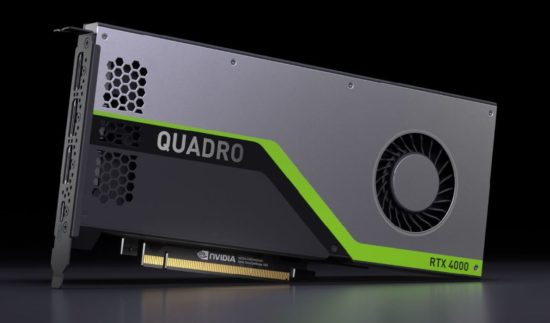
What Turing brings to Quadro
Nvidia had several metrics in mind for its performance goals for Turing, of course 3D graphics performance for traditional application usage, but also ray tracing and AI inferencing. Beyond the aforementioned aggregate increases in chip resources, Nvidia focused advancement efforts for Turing in four primary areas:
- An enhanced SM, including concurrent FP and INT and unified shared memory
- Advanced shading features
- Retained Tensor Cores, with optimizations for inferencing performance and performance/W
- New RT Cores, closing the gap on realtime ray tracing
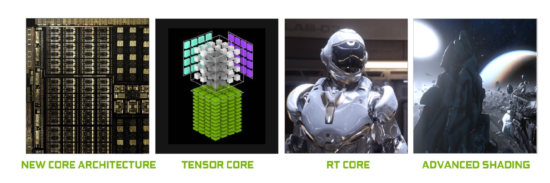
For a deeper analysis of Turing, with a closer look at Tensor and RT cores, check out https://gfxspeak.com/2018/10/25/the-tale-of-turing/.
Quadro RTX 4000 specifications
Succeeding the highly successful Pascal-generation Quadro P4000 in the workstation-class portfolio, the Quadro RTX 4000 hits a lower price point by leveraging a functionality-reduced version of the first-out-of-the-chute flagship TU102 chip that powers the new pair at the top end of the Quadro line, the RTX 8000 and RTX 6000. The RTX 4000’s TU106 contains around half the overall hardware resources of the TU102 (and there’s a TU104 in the middle driving the Quadro RTX 5000).
While the smaller TU104 (roughly 75% of a TU102) and the TU106 (around half) may drop a lower profile feature, both not only retain Turing’s signature features, Tensor Cores and RT Cores, they retain the same ratio of CUDA cores to Tensor Cores (8 per SM) and RT Cores (1 per SM). The TU102 and RTX 4000 shows that as Nvidia begins pushing Turing down the price and product spectrum, it is not de-emphasizing either AI inferencing or ray tracing, accelerated by those Tensor Cores and RT cores, respectively. No doubt, both represent capabilities Nvidia expects will best serve its long-term interests for new and established markets.
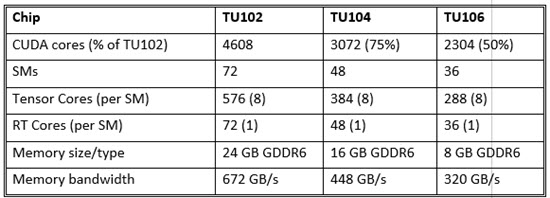
Paired with 8 GB of new GDDR6 memory (same footprint as the P4000), the single-slot RTX 4000 sells for about what the P4000 did at introduction (a sampling of on-line retailers reveals a street price today right around $1,075). Notably, at 160 watts max, the RTX 4000 consumes considerably more power than the 105 W P4000, though it stays within a single-slot width package.
How’s the Quadro RTX 4000 perform? First, the conventional comparisons with Viewperf …
As is our norm for evaluating workstation-class graphics cards, we ran SPEC’s Viewperf, in this case both the newest version 13 along with the previous version 12. Viewperf focuses workload on the graphics card, such that the rest of the system isn’t (or at least shouldn’t often be) the bottleneck. As a result, Viewperf will give a good idea of which card has the highest peak performance. However, it’s worth noting that the magnitude of any superior numbers does not indicate the level of superiority it will have in a real-world environment where the rest of the system, OS, and application may impose other bottlenecks.
For the purposes of comparison, we have previously tested Viewperf 12 results (circa Q1’17) of the Quadro P4000 running in the same Apexx 4 system (and with the most recent, published driver at that time). We also have Viewperf 13 results from our October 2018 testing of AMD’s natural rival to the Quadro 4000-class, the Vega-powered Radeon Pro WX 8200.
With this benchmarking exercise, we relied again on our standard testbench, an overclocked, liquid-cooled Apexx 4 workstation graciously loaned by Boxx. A high-performance platform like this one is desirable for running Viewperf in particular. Given the machine’s set-up, any bottlenecks that emerge can most likely be attributed to the graphics subsystem, rather than some other weak link in the system. Most important, we’re able to use it as a common testbench to compare multiple GPUs in a true apples-to-apples manner.
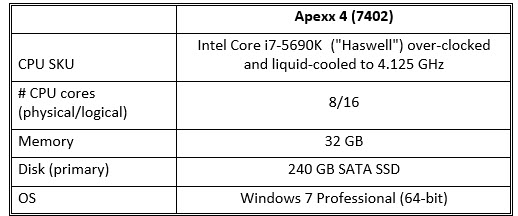
The upgrade story: the Quadro RTX 4000 versus its predecessor, the Quadro P4000
The Quadro RTX 4000 integrates about 50% more CUDA cores than its predecessor, the Quadro P4000, and benefits from commensurately higher bandwidth courtesy of GDDR6 technology. How does that translate to improved performance on Viewperf compared to its predecessor? In terms of raw scores, the Quadro RTX 4000 scored on average (across viewsets) around 30% higher than the P4000.
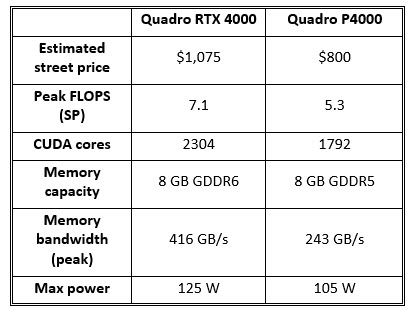
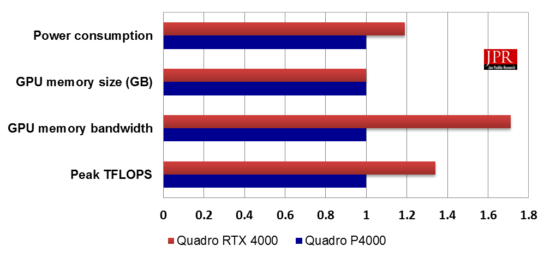
Factoring dollars and power, we find the RTX 4000 doesn’t perform as well in price–performance and performance/watt. Granted, the RTX 4000’s street price will likely drift down over time toward the P4000’s $875 level, the current (approximate) $1,075 yields price–performance on Viewperf 12 around 6% lower than the P4000. And given the RTX 4000’s higher power budget, performance/watt is about 9% higher than the P4000 managed on the same benchmark. (It’s worth noting that the RTX 4000’s specified max power is 160 W, however, 35 of those watts are allocated to the card’s VirtualLink/USB-C connector, rather than consume by the GPU itself.)
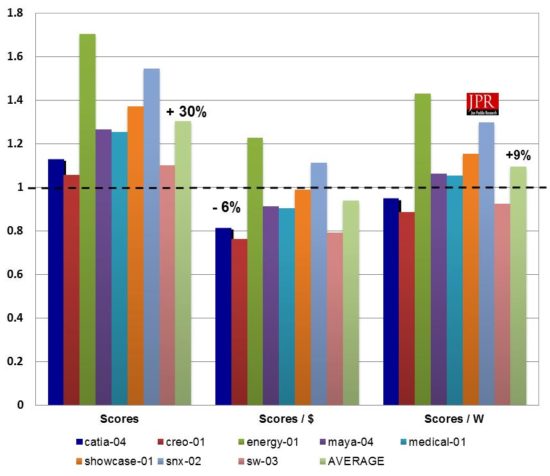
The competitive picture: the Quadro RTX 4000 versus AMD’s Radeon Pro WX 8200
Upon its introduction in the fall of 2018, AMD touted the Radeon Pro WX 8200 as the “world’s best workstation graphics performance for under $1,000.” AMD’s target with that claim was obvious: Nvidia’s lucrative Quadro P4000 product, which was launched in February of 2017 and had since dominated the just-below-$1K price point. The WX 8200 did perform well on Viewperf relative to the P4000, albeit with a downside of significantly higher power consumption and a dual-slot thickness. We also pointed out at the time that a P4000 successor based on Turing was likely in the offing, and now that it’s here, it’s worth revisiting relative Quadro RTX 4000 and Radeon Pro WX 8200 positioning at that $1,000-ish price point.
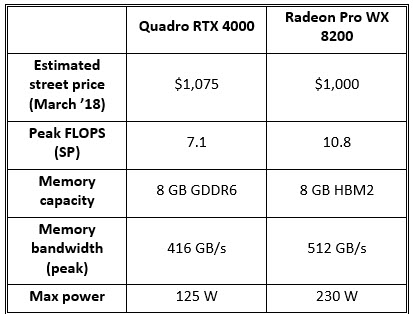
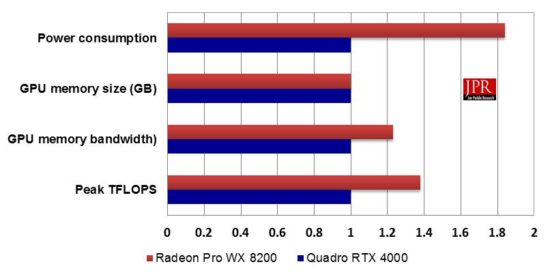
Using the more up-to-date Viewperf 13, the RTX 4000 managed to outperform AMD’s Radeon Pro WX 8200, with respect to raw scores, price–performance and performance/watt. The RTX 4000 posted 48% higher scores on average, while the WX 8200’s currently lower price point reduced the RTX 4000’s edge to around 38% on scores/dollar. Finally, the WX 8200’s notably high 230 watt rating pushed the RTX 4000’s advantage in scores/watt up to 173%. The RTX 4000 also has the advantage in form factor, maintaining the P4000’s single-slot thickness, compared to the WX 8200’s dual-slot width.
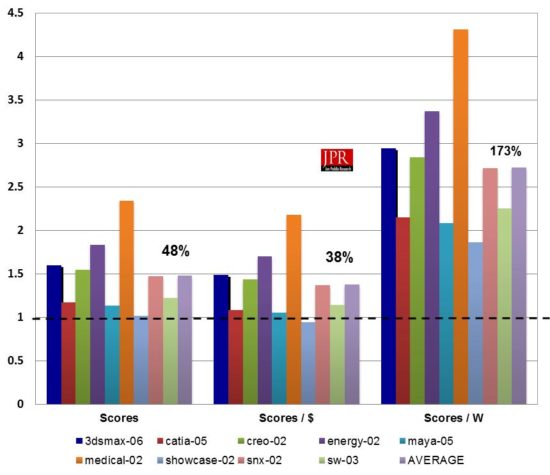
Assessing Turing-class GPUs requires an additional perspective
Viewperf is a fine benchmark. In fact, for past and current visual workloads in popular workstation applications, there isn’t a better tool for making comparisons. But again, with Turing, we’re no longer talking about hardware that’s outfitted just to accelerate traditional 3D graphics. With Turing, with the addition of Tensor Cores and RT Cores, Nvidia invested significant cost in functionality well beyond traditional 3D raster graphics, accelerating machine learning and ray tracing. In reality, even the machine learning hardware helps accelerate ray tracing through a deep neural network that de-noises the raytraced image while rays are still being fired, essentially taking a short cut to the final image. So the Tensor Cores are assisting the RT Cores in the aim of delivering on Nvidia’s claim of realtime ray tracing.
As such, whether you as a buyer have performance as a key criterion or not, it’s worth considering what the RTX 4000 can achieve in the non-traditional areas Nvidia chose to focus on: raytracing and machine learning. Let’s look at ray tracing first, as Nvidia looks to exploit not only the existing array of CUDA cores (as it has for its iRay renderer for several generations), but both the new RT Cores (for firing rays) and the Tensor Cores (for machine learning but directly contributing to ray tracing performance via de-noising).
While it will take some time to ramp the software infrastructure to support ray tracing pervasively (that goes for both professional visual applications as well as gaming), Nvidia is getting the ISVs and applications on board to get there. Earlier in support is Dassault with Solidworks, which has integrated RTX technology (leveraging the combination of Turing’s ray tracing acceleration, both in the form of Tensor and RT Cores) in its Visualize in-app renderer. Nvidia and Dassault demonstrated Visualize rendering four views of a 3D mechanical design in side-by-side windows, one with RTX technology enabled on the RTX 4000 and the other with it off. The window without RTX required 50% more time to complete. Inversely, that would mean an RTX 4000 leveraging newly integrated Turing ray tracing acceleration can run around 33% faster than without.
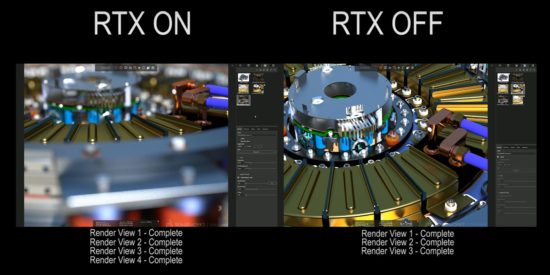
Remember, the RTX versus non-RTX gain is in addition to whatever speed up Turing can manage over Pascal without the help of RT and Tensor cores. Given the speed-up we saw for Viewperf (30%, which does not make use of those cores), then combining and extrapolating, it’s reasonable to expect the RTX 4000 to render about 70% faster (plus or minus of course, depending on specific scene) with RTX on over its predecessor, the P4000. Interestingly, that 70% is in the ballpark of what Nvidia also claims as the RTX 4000’s speedup over the P4000 on the rendering benchmark Luxmark 3.1. Based on the Nvidia-supplied chart, that speedup was in the 50–80% range, depending on scene. We didn’t run Luxmark, but based on our extrapolations, Nvidia’s figures would appear a credible speedup for the RTX 4000 over the P4000 on raytrace workloads, on average.
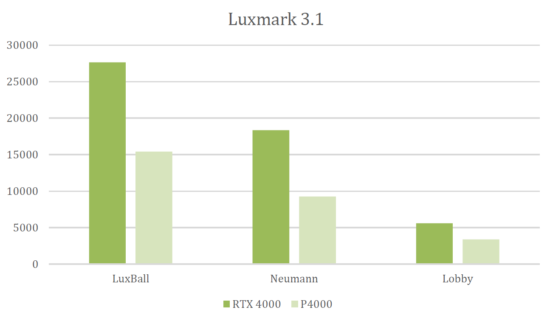
While the Tensor Cores help speed ray tracing through the use of a deep neural network (DNN) trained to de-noise a scene in mid-render, Nvidia research teams are finding and developing other uses for both machine learning and Tensor Core acceleration that dovetail nicely into professional visual workflows. Dubbed NGX, the company has formalized NGX, an enhanced set of DNN-driven image-enhancement features. I imagine Nvidia sees NGX today as anything but a fixed set of features, but rather an evolving and expanding toolbox of DNNs that can further harness machine learning for the benefit of Nvidia’s traditional visual markets. For Turing’s official launch, however, Nvidia’s pitching four specific NGX features, which will eventually be exposed to applications in the NGX API: Deep Learning Super Sampling (DLSS), AI InPainting, AI Super Rez and AI Slow-Mo.
DLSS is immediately valuable as a way to reduce the overhead of supersampled anti-aliasing by intelligently selecting fewer sample points, as opposed to more points of a conventional regular distribution. NGX’s other three advertised AI-enabled tools are geared more to quality enhancements for existing images and video, rather than those being created in realtime via the GPU’s graphics pipeline. AI InPainting uses Turing’s inferencing capabilities on a DNN (pre-trained from “a large set of real-world images” to essentially understand what’s missing (e.g., corrupted or intentionally deleted) in an image and fill in those areas with detail appropriate for the content.
AI Super Rez is similar to InPainting in the sense that a trained DNN is recognizing image content and filling in what isn’t there. Super Rez is employed during image scaling, which even with esoteric filters applied is subject to artifacts of aliasing, resulting in visual blocks or blurs. By contrast, Super Rez creates credible image data based on the content detected resulting in a more eye-pleasing result at virtually any scale factor. Finally, and also similarly, AI Slow-Mo creates intermediate video frames utilizing a DNN to intelligently interpolate video frames to create intermediate frames that didn’t otherwise exist, fitting the both content and temporal behavior.
While unfortunately not yet available to review, on paper these NGX tools should find an eager audience in one or more professional segments. The concept of Super Rez, for example, holds the potential to be a game changer for those in graphic design, who too often have to grapple with requests to blow up big images from low-res source files.
Nvidia’s willingness to sacrifice a bit of short-term discomfort for long-term gain has paid off before
Depending on what you’d anticipated seeing from the RTX 4000, the product will likely either meet expectations or disappoint.
If you expected a card that could achieve the level of improvement that its predecessor (the P4000) achieved over its previous generation (the Maxwell-based M4000), you may be disappointed. The Quadro RTX 4000 manages a meaningful but not stellar 30% improvement (averaged across viewsets) in Viewperf 12. In terms of price–performance, it delivers roughly the same scores/dollar (though that will likely improve over the product lifetime) as the P4000. And finally, it about 9% better performance/watt than the P4000. By contrast, the P4000 delivered a very impressive 87%, 82%, and 113% improvements, respectively, over the M4000.
But if you expected a card that delivers a meaningful bump in performance on existing visual workloads and provides a bridge to future workloads, then I’d say it’s succeeded. No, it doesn’t deliver dramatic gains on legacy usage over its predecessor. But it does fare very well compared to its primary market competition in terms of performance, power and form factor. And it would appear (both by benchmark data and logical extrapolation) that its speedup for ray tracing is far more significant. As such, it’s helping pave a path to a more ubiquitous ray tracing future, which I’d argue is more valuable to Nvidia long term, even if it means disappointing some today looking to run legacy visual workloads exclusively.
As with Fermi, Nvidia’s choice to lay groundwork for the future will likely pay off with Turing … though it may take some time
For the first time since Fermi, Nvidia’s Turing is a graphics-first chip with costly features that don’t directly serve Nvidia’s traditional market focus of 3D raster graphics, the type of graphics functionality that both games and professional visual applications have long relied upon. With Fermi, Nvidia was aggressively trying to make the transition to GPUs that could excel at more than graphics, to create a flagship new GPU architecture that could embark on the path of becoming more general-purpose compute accelerators for highly parallel, floating-point intensive applications.
In Fermi, Nvidia left some expensive functionality in its graphics-first chip/product SKUs—notably fast double-precision floating point and ECC memory—that helped the new applications it wanted to serve but did nothing for its bread-and-butter markets. As a result, Fermi’s performance/watt and performance/dollar suffered a bit in those markets. In exchange for that bit of suffering—which was not pronounced and proved short term—Nvidia achieved what it wanted to: establishing a foothold in HPC applications and datacenters which has since blossomed into a $3 billion-plus dollar business with its Tesla brand GPUs.
With Turing, Nvidia’s strategy isn’t so different, using a new generation to provide a bridge to new client-side applications and functionality, namely AI and ray-tracing. One laudable difference with Turing compared to Fermi is that Nvidia has developed ways to leverage Turing’s AI hardware (Tensor Cores) to improve traditional 3D graphics with applied features like Deep Learning Super Sampling (DLSS). Still, sacrificing significant cost in Turing to include both sets of new cores reflected a gamble we believe Nvidia was very consciously making.
Will the demand for realtime ray tracing emerge in dramatic fashion—and therefore justify the choices Nvidia made with Turing—the way non-graphics compute applications did? There’s no guarantee, but I’d argue that long-term, pursuing ray tracing with Turing is a safer bet than pursuing HPC with Fermi and subsequent generations. I’d further make the case that eventually, all 3D visual processing which has photorealism as a goal will be ray traced. While the industry has cleverly and consistently improved upon the 3D raster model, it’s ultimately still a hack when it comes to global illumination … and ray tracing will always do better where photorealism is the goal.
Of course, the big question from that previous statement is how long eventually will be, as a flip from traditional OpenGL/DX raster graphics to ray tracing will be anything be quick. Though they both produce 3D images, ray tracing is a fundamentally different algorithm requiring a different architectural approach to implement optimally. And that means to make the transition to ray tracing will not only take quite a bit of time, it will mean having to break the chicken-and-egg dilemma software and content developers face: why spend a lot of time or money on ray tracing when the installed base of hardware isn’t very good at it yet? That’s where the long-term value of Turing and Quadro RTX lies, to help break that Catch-22 and help kickstart a mass-market transition. The transition has to begin somewhere, and this looks a viable time to start.
By that definition, Nvidia will likely achieve success with Turing. Not because it has introduced a product that’s a slam-dunk for existing usage and applications. Rather, because the company has once again taken a gamble to produce hardware to help seed a transition to new usage models and applications, knowing the payoff is likely a long term one. That’s what happened with CUDA and the compute-focused features Fermi introduced, again eventually. Nvidia’s bet on GPU-compute opened up markets and opportunities precisely the way the company had hoped, even if the transition wasn’t as smooth as it might have planned at the outset.
Until more momentum develops, however, Nvidia will have to weather some criticism just as it did with the transition to Fermi. With Fermi, much of that criticism centered around power consumption and thermals. In the case of the Turing Quadros (and GeForces for that matter), the criticism is that they don’t deliver quite the inter-generational punch they should for conventional 3D graphics. There’s some truth to that, and certainly the 4000 RTX can’t match the jump on Viewperf that the P4000 managed before it. Still, the 4000 RTX should do fine, even when measured in conventional terms. It represents a capable if not stellar upgrade in “legacy” usage models, and more importantly, it can argue superiority over its primary market competition.
What do we think?
I appreciate a strategy that takes calculated risks to pave a path all logic says we’re headed to eventually, even if the reward is further out than usual short-scope decision making allows. Granted, that’s the sentiment of an analyst, not an investor or a GPU shopper, who might feel differently. But looking back years from now, chances are the criticism and RTX’s more mundane improvements in legacy 3D graphics will be forgotten. And it’s altogether possible we’ll judge the Turing family as the linchpin that started the transition to the era of ubiquitous ray tracing. And if that’s true, then Nvidia will be more than happy to have weathered some short-term discomfort to have pioneered that shift.