Neural Haircut is a novel approach proposed by researchers from the Samsung AI Center and Friedrich-Alexander-Universitat. The method aims to accurately reconstruct hair geometry at a strand level from either monocular video or multi-view images, even when captured in uncontrolled lighting conditions. The main idea behind the strand-based hair reconstruction is to use geometry texture and a pre-trained strand parametric model on synthetic data. It is applicable to both monocular video and multi-view image inputs, making it versatile and effective for various hair reconstruction tasks.
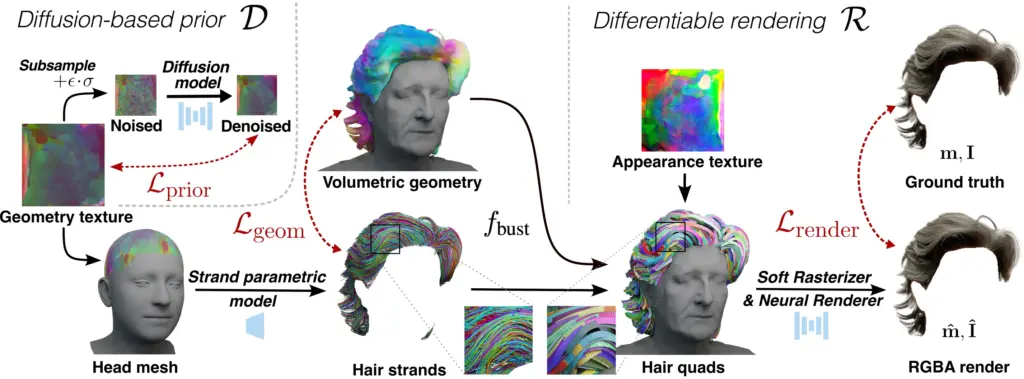
The reconstruction process consists of two main stages. In the first stage, the approach jointly reconstructs coarse hair and bust shapes as well as hair orientation using implicit volumetric representations. The use of implicit volumetric representations allows capturing the overall hair geometry and orientation from the input data.
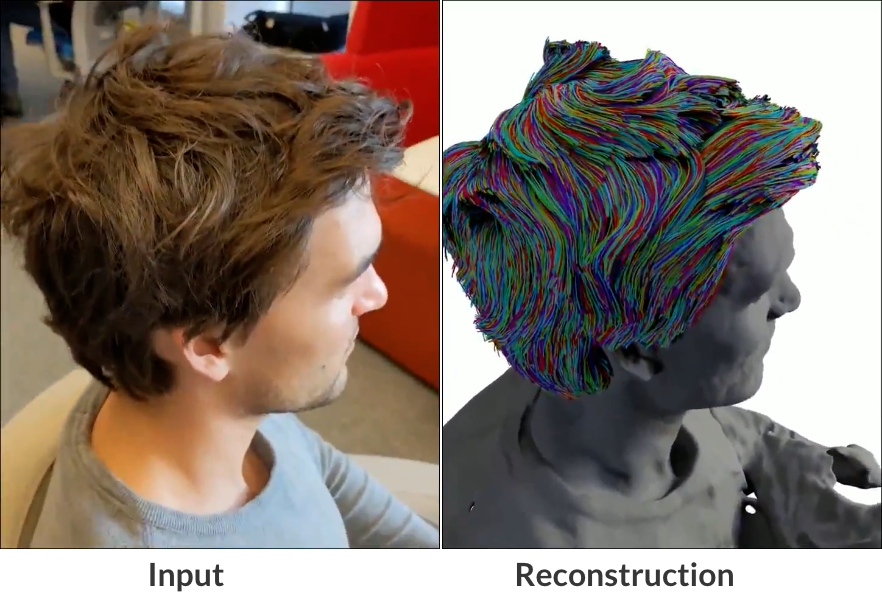
The second stage focuses on estimating a strand-level hair reconstruction. This is achieved by leveraging hair strand and hairstyle priors learned from synthetic data. These priors guide the reconstruction process to ensure more realistic and personalized hairstyles. To further enhance the reconstruction fidelity, the approach incorporates image-based losses into the fitting process using a novel differentiable renderer.
The main idea behind the strand-based hair reconstruction is to use geometry texture and a pre-trained strand parametric model on synthetic data. At each iteration, a set of random embeddings is sampled from the texture, and corresponding hair strands are generated using the pre-trained model. These strands are then supervised using geometric and rendering-based constraints.
The geometric loss ensures that the reconstructed hair strands adhere to the volumetric reconstruction and maintain proper orientations. The silhouette-based and neural rendering losses utilize a soft hair rasterization technique based on hair quads with learnable strand-based appearance texture. This allows predicting the silhouette and RGB image of the reconstructed hair. A regularization penalty is introduced to improve the physical plausibility of the obtained strands. This penalty is based on a pre-trained diffusion model on synthetic hairstyles.
Reference
Sklyarova, V., Chelishev, J., Dogaru, A., Medvedev, I., Lempitsky, V., & Zakharov, E. (2023). Neural Haircut: Prior-Guided Strand-Based Hair Reconstruction (arXiv:2306.05872). arXiv. https://doi.org/10.48550/arXiv.2306.05872