Researchers have introduced Objaverse-XL, a groundbreaking web-crawled dataset of 3D assets. With an astonishing scale and diversity, this dataset comprises over 10 million 3D objects, revolutionizing the capabilities of state-of-the-art 3D models. The Objaverse-XL dataset addresses the long-standing challenge of data scarcity in 3D computer vision, offering a rich variety and quality of assets sourced from diverse locations on the Internet.
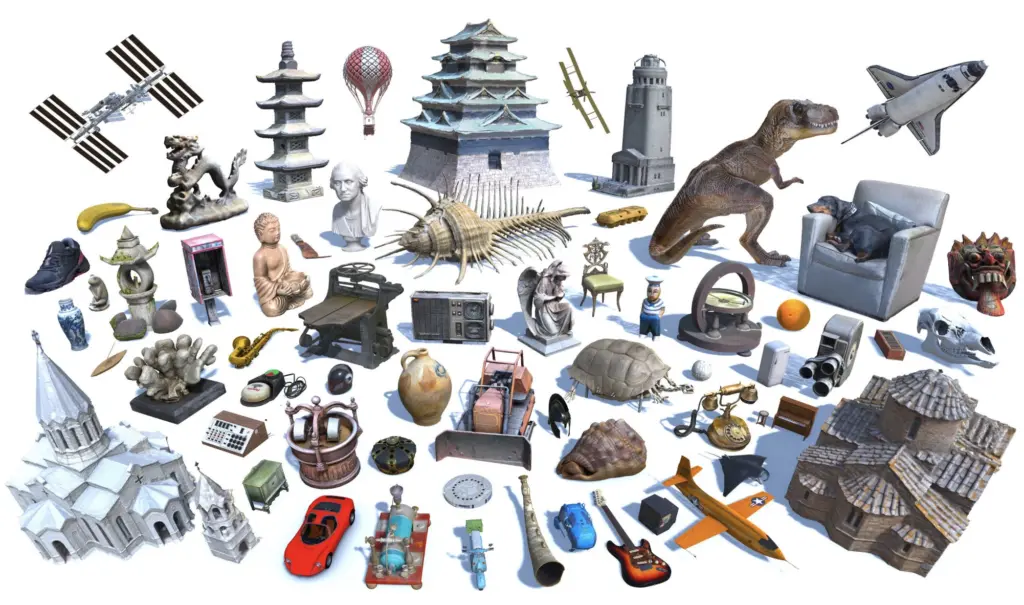
Traditional methods of 3D data collection have relied on small handcrafted datasets, hindering progress in applications like 3D object generation and reconstruction. Limited by expensive software and professional 3D designers, the scarcity of data has become a bottleneck for learning-driven approaches. However, Objaverse-XL marks a turning point by leveraging advances in 3D authoring tools, photogrammetry, and the abundance of 3D data available online.
This expansive dataset scours numerous online sources, including software hosting services like Github, specialized 3D asset platforms like Sketchfab, 3D printing asset sources such as Thingiverse, 3D scanning platforms like Polycam, and even repositories like the Smithsonian Institute. By aggregating data from these diverse sources, Objaverse-XL provides an unprecedented resource for training and benchmarking 3D models, surpassing previous efforts like Objaverse 1.0 and dwarfing the scale of ShapeNet by two orders of magnitude.
The impact of Objaverse-XL on 3D computer vision is already evident. State-of-the-art models, such as Zero123 for novel view synthesis and PixelNerf for synthesizing novel views from a small set of images, demonstrate remarkable improvements when pre-trained with Objaverse-XL. These models showcase enhanced zero-shot generalization to a wide range of complex modalities, including photorealistic assets, cartoons, drawings, and sketches. Moreover, the benefits of scaling pre-training data from a thousand assets to 10 million assets are evident, with continued improvements and untapped potential emerging from the vastness of web-scale data.
The introduction of Objaverse-XL not only addresses the current limitations in 3D object generation but also paves the way for the increasing demand and interest in augmented reality (AR) and virtual reality (VR) technologies. As these technologies continue to grow, scaling up 3D data becomes crucial, and Objaverse-XL stands as a vital resource to drive innovation in this field.
With its unparalleled scale, diversity, and access to millions of 3D objects, Objaverse-XL represents a significant milestone in 3D computer vision. This new dataset empowers researchers and practitioners to push the boundaries of what is achievable in 3D modeling, unlocking a world of possibilities for applications ranging from virtual environments to immersive experiences.
While it is much larger than its predecessor, Objaverse 1.0, the new database still falls short compared to modern billion-scale image-text datasets. To further advance the field, researchers suggest future work should focus on scaling 3D datasets and making 3D content easier to capture and create. Additionally, not all samples in Objaverse-XL may be necessary for training high-performance models, so selecting representative datapoints becomes crucial. While the current focus is on generative tasks like novel view synthesis, future exploration should consider how Objaverse-XL can be applied to discriminative tasks such as 3D segmentation and detection, potentially enhancing their performance with the rich and diverse dataset.
Reference
Deitke, M., Liu, R., Wallingford, M., Ngo, H., Michel, O., Kusupati, A., Fan, A., Laforte, C., Voleti, V., Gadre, S. Y., VanderBilt, E., Kembhavi, A., Vondrick, C., Gkioxari, G., Ehsani, K., Schmidt, L., & Farhadi, A. (2023). Objaverse-XL: A Universe of 10M+ 3D Objects (arXiv:2307.05663). arXiv. https://doi.org/10.48550/arXiv.2307.05663