A scattershot view of the latest news on the front
Looks like the first quarter of 2018 is shaping up to be big for the future of AI. Companies in the segment including cloud companies, IP companies, and the traditional semiconductor companies all have major ambitions for the huge explosive market that AI is becoming.
A battle is forming around processor platforms, cloud companies Google favoring custom chips to augment classic semiconductors such as CPUs and GPUs. Semiconductor and IP companies are designing chips to enable efficient hardware and neural net systems that can run faster and use less power. Intel, on the other hand is proposing its open platform ecosystem built on Xeon, FPGAs, and specialized processors including Nervana and Saffron.
Cloud companies opt in
Google has recently announced the availability of its Tensor Processing Unit (TPU), which will be accessible via the Google Cloud. Google introduced its TPU at Google I/O last year. At that time the chip was being used by beta customers. The company’s high-profile beta customer at the time was Lyft, which was using AI to recognize surroundings, locations, street signs etc.
Just in time for Valentine’s day, Google announced the availability of its TPUs for public beta. The company also offers test projects for customers to play around with as well as allowing custom projects. Developers interested in trying out Google TPUs must apply for time and tell Google what they want to do with the system. The pricing for a Google TPU is $6.50/hour. As TechCrunch has noted, Nvidia’s GPU option runs $1.60/hour, but that’s where the power comparison comes in. The peak performance of a single TPU board is 180 teraflops compared to 21 Teraflops for the Tesla P 100.
The cloud-based TPU features four ASICS with 64 GB of high bandwidth memory.
Nvidia
Nvidia has announced its new Volta GPU with 640 tensor cores, which delivers over 100 Teraflops, has been adopted by all the leading cloud suppliers including Amazon Web Services, Microsoft Azure, Google Compute Platform, Oracle Cloud, Baidu Cloud, Alibaba Cloud, Tencent Cloud and others. On the OEM side, Dell EMC, Hewlett Packard Enterprise, Huawei, IBM and Lenovo have all announced Volta-based offerings for their customers.
Microsoft and Intel
Microsoft has teamed with Intel and is offering FPGAs for AI processing on Azure. FPGAs are currently being put to work on Microsoft’s in-house learning operations as well as providing Azure customers with accelerated networking and compute. Microsoft says they will provide customers the ability the run their own models on the FPGAs.
With the acquisition of FPGA maker Altera in 2015, Intel admitted that the X86 architecture can’t do everything. Over the years, Intel has had faced these facts of life over and over again, only to get it wrong and retreat back to their X86 forever stance, but it’s different this time as the entire tech industry comes to grips with the end of the PC era and Intel’s CEO Krzanich represents the return of the engineer as an Intel leader. Intel sees FPGAs as the perfect companion to its processors for AI work.
AI, in fact, has brought the FPGA back to life as tool of innovation rather than a back-room development platform. Its very flexibility and adaptability fits in with AI tasks. Intel has put all its manufacturing might, packaging know-how, and high dollar components to work to build the new Stratix X FPGA as a partner to Intel’s Xeons.
Last August at Hot Chips it was revealed that Microsoft has partnered with Intel on Project Brainwave for Azure. Intel’s 14 nm Stratix 10 FPGAS accelerate Microsoft’s Azure-based deep learning platform. (MS announced in 2016 it would use FPGAs for AI.) Microsoft claimed at Hot Chips that its use of FPGAs with “soft” Deep Neural Network (DNN) Unit synthesized onto the FPGAs instead of hardwired Processing Units (DPUs) is an advantage even though DPUs might be faster. Microsoft says they have defined highly customized, narrow precision data types that increase performance without losing model accuracy and because Project Brainwave can scale across a range of data types by changing the software DNN according to requirements. In contrast, DPUs are designed for specific operators and data types. Microsoft argues that this kind of flexibility makes sense in a field as fast moving as AI. Microsoft says it can incorporate research innovations into the hardware within a few weeks. (For more detail and probably a better explanation, see Microsoft’s discussion or HPC Wire.) Brainwave is designed for live data streams including video, sensor feeds, and search queries.
Here is a list of AI processors.
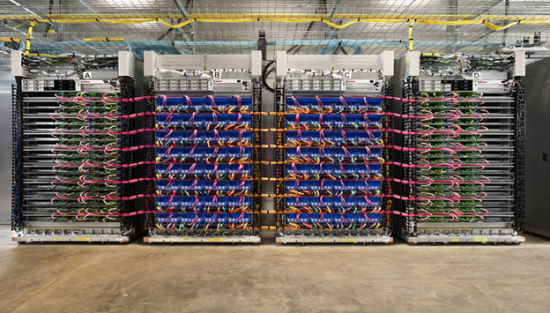
Amazon’s AWS also relies on off the shelf processors including Intel’s Xeons, Xilinx FPGAs, and also GPUs. The company gives customers the ability to sign up for the best combination of processors for the job. The strength of AWS is its ability to package complexity and sell it. AWS has also been encouraging developers to initiate DIY machine learning projects using the company’s Sagemaker machine learning system to build, train, and deploy machine learning models. Lately, the company has introduced the Deep Lens camera with built-in deep learning algorithms for image recognition that developers can use to explore deep learning with Sagemaker.
At introduction AWS CEO Andy Jassy demonstrated how to build a music recommendation service based on algorithms and optimized with Sagemaker and also how to add visual data using the new Deep Lens camera on sale at Amazon for $249. He said that the process of creating and putting models to work is so easy with Sagemaker, he expects to see people play with the system over and over again. Amazon says some of the use models that Sagemaker has been optimized for including built-in capabilities include tools for ad targeting, credit default prediction, industrial IoT and machine learning, supply chain and demand forecasting, and click through prediction. Sagemaker also supports industry frameworks, and enables developers to create their own.
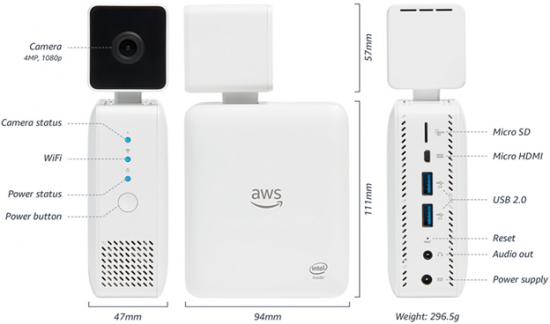
Saffron was established in 1999 by two researchers working in IBM’s Intelligent Agent Lab in Raleigh NC, not long after the so-called “AI winter” of the mid-80s when enthusiasm for AI dramatically fell off as processors at the time seemed inadequate to mimic human intelligence. Nevertheless, the Saffron team continued development to do just that and Intel acquired the company in 2015. Saffron’s bedrock technology is the Natural Intelligence Platform (NIP). In true Intel fashion, Intel has looked at custom chip development, GPUs, FPGAs, and CPUs and has set out to define the base hardware architecture for AI. Intel has multiple processor options for AI, including Xeon, FPGAs, Nervana, Movidius, and Saffron.
Intel has beefed up its Xeon Processors for AI and HPC including features for performance and interconnectivity. The Advanced Vector Extension 512 (Intel AVX-512) improves performance and throughput for advanced analytics, HPC applications and data compression QuickAssist Technology speeds up data compression and cryptography and of course Intel continues to improve its hardware security.
In August 2016, Intel announced it had also acquired Nervana, a startup that has been developing artificial intelligence software and hardware for machine learning. It October 2017, Intel unveiled the Nervana Neural Network Processor (NNP) formerly known as “Lake Crest.” Nervana has been working on the chip, which is designed expressly for AI and deep learning, for over three years. For Intel, the beauty of Nervana is that it has been designed and built from the ground up expressly for machine learning. Intel VP and GM for the artificial intelligence products group says in a blog note, “We designed the Intel Nervana NNP to free us from the limitations imposed by existing hardware, which wasn’t explicitly designed for AI.”
Intel plans to deliver chips to a few partners this year, including Facebook. Intel said they collaborate closely with large customers to determine the set of features they needed. Early next year, customers will be able to build solutions and deploy them via the Nervana Cloud, a platform-as-a-service (PaaS) powered by Nervana technology. And larger organizatons will be able to use the Nervana Deep Learning appliance, which is effectively the Nervana Cloud on premise.
Intel acquired Movidius in 2016 to get VPU technology, a missing link in its arsenal for machine learning and AI. It has formed an important segment in Intel’s NTG group led by SVP and GM Josh Walden. The group includes Intel Labs, perceptual computing, the new business group including wearables, sensors, etc., the maker and innovation group and Saffron, which Walden describes as “the reasoning” part of Intel’s AI products. The group takes a long view—at least ten years out says Waldon.
Intel’s Movidius devices include a Neural Compute Stick, the Myriad 2 and the Myriad X is an SoC, which combines dedicated imaging, computer vision processing, and an integrated neural compute engine. The chip can power intelligent and autonomous devices such as robots, drones, security cameras, automotive devices, etc. We’ve talked about it quite a bit in our VPU stories and our VPU report.
Apple
The best known mobile AI processor is included with the latest Apple iPhone X and so far, it hasn’t blown anyone away with its brilliance unless you consider talking emoji puppets (animoji) using face tracking to mimic your speech the sine qua non of intelligence. As a reminder, Apple’s A11 is a 64-bit ARMv8 six core CPU with two high performance 2.39 GHz cores called Monsoon, and four energy efficient cores, call Mistral. The A11’s performance controller gives the chip access to all six cores simultaneously. The A11 has three-core GPU by Apple, the M11 motion coprocessor an image processor supporting computational photography, and the new Neural Engine that comes into play for Face ID, Animoji and other machine learning tasks. Our request to buy an iPhone X for test was received with gales of laughter so we can’t speak for ourselves, but we could find no review, report, or anecdotal evidence that Apple’s AI has done one thing to improve Suri’s insane conversational leaps.
It is our belief that the iPhone X is Apple’s initial flyer for a phone with AI smarts at the edge and that we’ll see considerable improvement in future products. If not, the competition is getting smarter faster.
Arm
Arm has been threatening to join the AI party and has been putting together the pieces starting with its 2016 acquisition of Apical for imaging technology. At the IEEE conference Arm discussed its Trillium Platform which includes Machine Learning (ML) and Object Detection (OD) processors complementing Arm NN Software, and the existing Arm Compute library and the CMSIS-NN Neural Network kernels. Arm describes the software as providing a bridge between popular NN training frameworks and the Arm-based inferencing implementations. (See VPU report, Q3’17, page 38.)
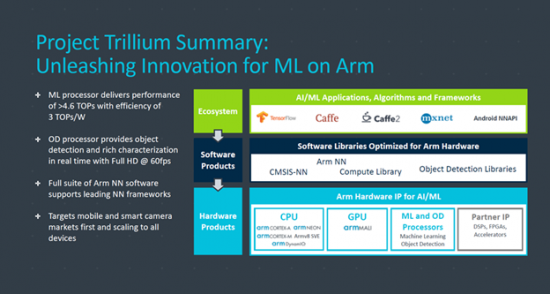
Amazon
According to a story in the Information, Amazon is reportedly planning on building hardware accelerated AI into Alexa, so she can respond faster and smarter. As it is now, Alexa has to pass everything to the cloud and back. If the Internet is down, she’s as dumb as a rock. Also, if there is at least some local AI processing Alexa can have a better idea of what’s going on in the house when the question is asked so she won’t necessarily react to false positives and could be more discerning about what goes up to the cloud. It’s been suggested that more local processing will reassure those concerned with Alexa’s constant communication with the cloud. (We’re doubt it.) Currently, the Echo Show relies on an Intel Atom and the Echo Dot has TI processors inside. Amazon’s AWS makes Xilinx FGPAs available for cloud-based processing for Alexa.
Huawei
Huawei has announced that its latest mobile processor, the Kirin 970, will feature a neural engine. The new chip will have an 8-core Arm-based CPU, 12 Core GPU (Mali), an Image DSP, and the Kirin Neural Processor supporting 1.927 TFLOPS at 16-bit floating point. Huawei says the NPU can perform AI tasks 25× to 50× faster and with less power.
Qualcomm
When it comes to mobile semiconductors, Qualcomm, as always, is the big gorilla and as of this writing the gorilla has a new lease on life, now that US courts have moved to investigate the hostile merger initiated by Singapore-based Broadcom.
Last December at a company meeting for press and analysts Gary Brotman, Qualcomm’s head of artificial intelligence and machine learning product management introduced the Snapdragon 845, which would later get a big rollout at CES and Mobile World Congress in Barcelona. He said “the Snapdragon 845 is our third generation AI platform.” The chip provides AI features on the phone because, says Brotman, “you shouldn’t have to be connected to the Internet to take advantage of the intelligence in your device.”
The Snapdragon’s Neural Processor Engine takes advantage of the hardware enhancements for AI built into the 845 as shown in the accompanying diagram. The chip is well equipped to take on Apple’s A11 with support for facial recognition, photo effects including background bokeh, and a more personal interaction with the phone.
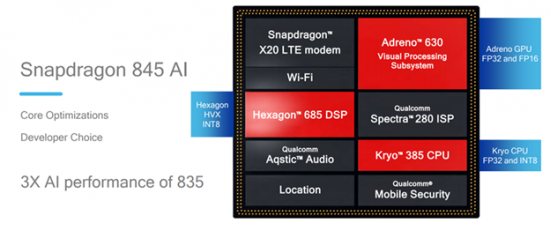
The mobile AI market is going to change lives. The AI processing at the edge isn’t just for mobile phones, it’s for the whole world. It will go into home devices, surveillance systems, automobiles, airplanes, glasses, watches, and probably tennis shoes. And as all vendors mentioned in this story and those I missed (most notably IBM) build and sell systems and their customers built and deploy models and eventually standards evolve for selling and sharing models a sea of data is filling up.
What do we think?
It might be snowing on the east coast of the U.S. and there are snowball fights going on at Rome’s Coliseum, but it’s Springtime for AI all over the world. There is some of this work that can make one a teensy bit queasy and there are world powers jockeying for data supremacy. Much of this stuff has not been worked out and truly, we just don’t know the long-range effects. We could suffer full scale invasion of our intelligence systems, in fact, it’s being argued that we already have.
We have to believe that more innovation, and open platforms, widely available access to tools for everyone, has to be positive trends but the stakes are probably so much higher than we currently realize.