In a recently published paper, researchers in China introduced DreamEditor, a new approach to editing neural fields with text prompts. DreamEditor uses a mesh-based neural field for scene representation. This approach enables localized editing within specified regions, providing greater accuracy and flexibility. By harnessing the capabilities of a pre-trained text-to-Image diffusion model’s text encoder, DreamEditor can autonomously identify areas for editing based on text prompt semantics.
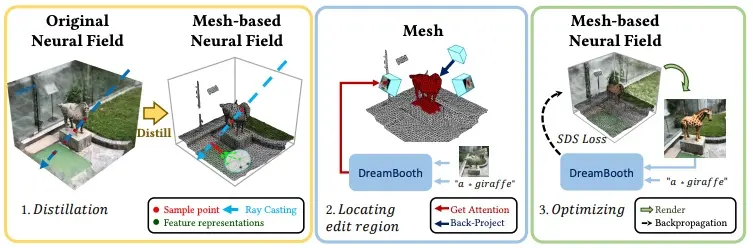
The system further optimizes the editing region by aligning its texture and geometry with the provided text prompts. This is achieved through the application of score distillation sampling, a method referred to in previous research.
The researchers says that extensive experiments have shown DreamEditor’s effectiveness in accurately editing neural fields in real-world scenes as per given text prompts. This technique also ensures consistency in areas not relevant to the edits. The resulting textures and geometry generated by DreamEditor are highly realistic and outperform preceding methods in both quantitative and qualitative evaluations.
DreamEditor’s design allows for various editing effects such as re-texturing, object replacement, and object insertion. The use of a mesh-based neural field in this framework converts 2D editing masks into 3D editing regions. This process disentangles geometry and texture, thus reducing the risk of excessive deformation.
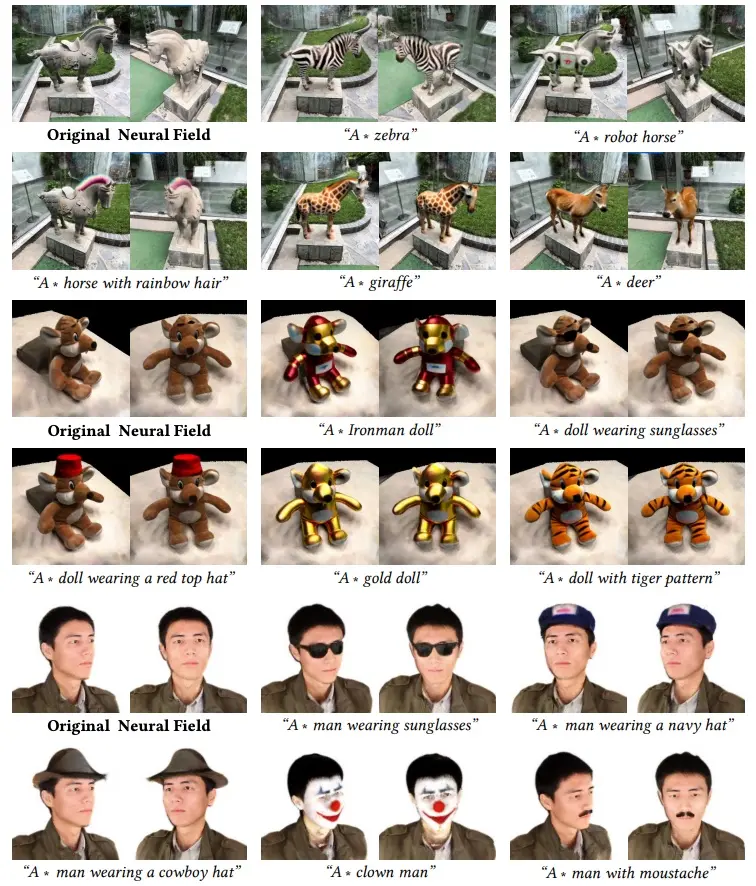
The innovative three-stage process of DreamEditor includes transforming the original neural radiance field into a mesh-based neural field, employing a Text-to-Image model trained on the specific scene, and applying the edited modifications to the target object within the neural field.
By ensuring high levels of fidelity and realism, DreamEditor paves the way for more precise and intuitive 3D scene editing. This development holds significant implications for industries utilizing scene reconstruction and view synthesis.
Reference
Zhuang, J., Wang, C., Liu, L., Lin, L., & Li, G. (2023). DreamEditor: Text-Driven 3D Scene Editing with Neural Fields. ArXiv Preprint ArXiv:2306.13455. https://doi.org/10.48550/arXiv.2306.13455