In 2007 Collaborative Product Development Associates completed an in-depth study of how enterprise manufacturers were taking advantage of virtual product simulation. Analyst Michel Vrinat explains the firm’s findings. From the February 2007 edition of Engineering Automation Report, acquired in 2010 by Jon Peddie Research.
By Michel Vrinat
Collaborative Product Development Associates
Engineering Automation Report, February 2007—Given the major benefits attributed to virtual product simulation, and the rapid growth in the analysis/simulation market, Collaborative Product Development Associates, LLC (CPDA) recently decided it was time to assess the current status of leading edge users and gating factors for success. CPDA recently completed a study across industry verticals to assess the level of maturity of companies with respect to simulation on six key issues, raised as priorities by users. Twenty companies, primarily in the USA, participated. The effort is currently being extended systematically to Europe and Japan. In-depth interviews with key representatives of each company in the simulation and engineering area were conducted. For each of the six categories, CPDA established a scorecard with five levels of maturity.
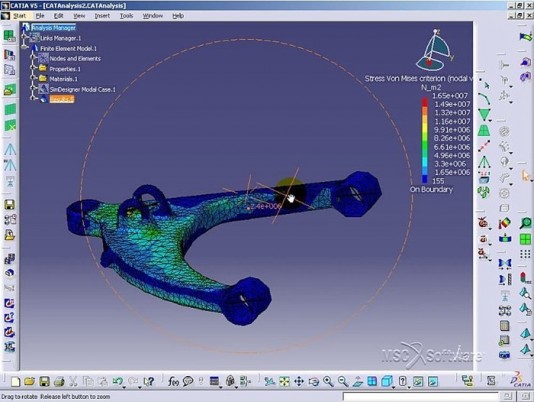
The results show strong progress in the use of simulation across multiple disciplines over the recent past, reflecting the growth in software and services in CAE. Moreover, many companies have established leading edge development efforts across many of the areas investigated. However, these same efforts are far from complete, and have not yet been implemented broadly across operations. Indeed, application so far has been highly inconsistent across the firm, and across sectors.
A wide range in maturity ratings results across industries companies and—several times—even within the same company from different groups. This wide range clearly reflects differences of opinion in evaluating internal maturity levels as well as the actual or real differences in deployment of the most advanced concepts such as multi-disciplinary analysis, abstract data modeling, or the linkage and reconciliation of performance results with requirements. Comparing your own company’s efforts against these results will give you feedback on areas where your company trails, and those where you may have gained competitive leadership.
Automotive leads the pack
In general, automotive led aerospace across four of the six categories reviewed as seen in the accompanying chart. Consumer Packaged Goods (CPG) trailed slightly in a number of categories. The automotive sector has automated many procedures and made them available to mainstream engineers. The motivation to automate simulation processes within the automotive industry may be higher because of the recurring analysis applied to many similar product models, which justifies the investment. The aerospace industry tends to develop new methodologies and tools for a project because of the rapid evolution of technology between programs, the availability of new materials, new concepts in the structure, and changes in the certification processes. Therefore, each aerospace program has to develop new methods and sometimes tools to support simulation in many disciplines. That leaves less time and requires more investment to automate processes, precluding high potential for return from mainstream engineering.
Category 1: Requirements management and performance verification
Requirements management across product development supports “right to market” objectives, and avoids under- or over-designed products, with better quality and cost optimization. The management of customer or market requirements begins with initial system design in the conceptual phase, and continues through detailed engineering with the release to production. This requires a deep level of detail in order to drive design decisions and verify product performance against those requirements.
The approaches taken range from manual, paper-based processes through fully mature, bidirectional reconciliation of requirements with product performance metrics. This area represented the lowest level of maturity, or achievement, in the study with an overall average of 2.0 on a five-point scale, despite some progress with system engineering practices and requirements management tools. The weakness appears with the lack of detail for requirements flow down, which does not consistently reach a level directly usable by engineering. Moreover, the link between requirements management and performance verification is often inadequate, or even non-existent.
Category 2: Knowledge capture and reuse, standard work procedures
Capturing the knowledge of experts in simulation presents a tough job because all too often the experts feel threatened with their job at risk, or they simply lack the time or interest to contribute. Their stance can make it extremely difficult to capture their experience and make it re-usable and accessible to any engineer. The pay-off, however, would make simulation a mainstream activity, leading to better quality and reduced cycle time.
The companies participating attained an average overall maturity level of 2.4. Clear progress has been achieved over the last several years. Indeed, four companies reach level four in maturity as characterized by automated assembly of analysis models and execution based on standard procedures, and rules and templates. Even so, the range of results varied dramatically with six companies rated at the base level 1 maturity supporting only ad hoc work procedures based on individual experience.
Category 3: Multi-disciplinary integration
What is the actual priority and need for a common data model across disciplines, such as NVH, crash, structural or aerodynamics? How are multi-disciplinary analyses supported? Those questions should be asked and addressed systematically in the industry, because of the increase in the need for multi-disciplinary analysis to optimize product performance and to meet the constraints on innovation such as those associated with composite materials and interactions across disciplines. The pay-off is to avoid engineering re-work and the waste of IT resources, to increase product quality and robustness, and to reduce the overall development cycle time and production costs. Indeed, cycle time and costs represent the two most critical challenges any company faces today.
The definition of multi-disciplinary analysis differs across the industry and sometimes even within the same company. It directly depends on the phase of the development project with requirements that vary significantly from system design to detailed engineering. The specific disciplines involved such as structural, NVH, or aero-acoustic also impacts the approach.
Multi-disciplinary integration issues largely reflect the organization of the company. Isolated groups of experts hold responsibility for particular types of simulation and frequently use different tools, different sets of data and varying methodologies. Finding a common ground and rationalizing simulation presents challenging tasks.
Given the repetitive simulation required across product variants, automotive has pushed aggressively to link specific disciplines. For some companies, a tight coupling between simulation disciplines such as structural and thermal has been achieved with great success and complete integration in an automated environment.
Outside automotive, the potential for a common data model to serve multiple disciplines ranks low in priorities across industries, except for relatively narrow applications serving a limited number of tightly coupled disciplines. The potential for an abstract model to drive design and simulation from the conceptual phase on across the full design cycle appears to rank even lower. On a positive note, the need for a common simulation framework for application integration is currently under study at many these companies and some have already applied it partially or piloted an effort.
Category 4: Performance data repository for feedback on performance from simulation, test, and operations
Volumes of data should feed back to development from multiple sources such as simulation, test and field operations. Reconciling and managing the data supports a dramatic payoff. Performance or quality may be boosted based on results already available from the results of a previous project at minimal cost with the full integration of simulation, test and operational data.
Predicting system or parts behavior depends on mathematical models that need to be validated by physical tests to tune the simulation parameters and select the proper solver. When the product hits the road or starts test flights, a great deal of operational data can be collected and used to further validate the performance predictions, especially for durability, fatigue or complex interaction effects. Therefore, the ability to access the data for comparison with simulation results represents a key enabler for better quality, maintenance cost reduction and follow-on project development.
Unfortunately, industry registers a relatively low score of 2.2 on average in this area, with automotive again clearly leading aerospace. This is a concern because of the impact on product design quality and the inability to re-use past project experience that can greatly reduce product development time.
Category 5: Waste reduction, DFSS and lean design
What are the current practices to reduce the waste of time and materials? What is the current practice to support lean design, set based design, and Design for Six Sigma (DFSS)? As a strategic initiative supported by daily follow up, the pay-off not only reduces the waste of time and resources, but leads to higher quality and to fewer defects as well.
The participants achieved the second best average level in this area. Again here, the automotive sector drives well ahead of aerospace, despite a clear lead for the aerospace industry in actually achieving quality. There is a strong motivation in automotive for DFSS as applied to engineering and lean design. Despite the effort, questions still come up concerning the efficiency of the methods being applied given the continuing challenges concerning quality, which U.S. Automotive faces today. As one writer provocatively stated, quality is what you talk about when you do not have it.
In both automotive and aerospace, DFSS represents a strategic objective, generally followed very closely by management. Methods are standardized and documented for re-use. Performance metrics are in place in a number of cases.
Lean design is not as heavily emphasized in the automotive sector. In the aerospace industry, the contribution of simulation to reach lean design objectives is clear, especially during conceptual phases where the main design decisions are taken. Lean is not a general concern in the consumer products area.
Finally the concept of set-based design is generally not known and almost never applied. Several companies mentioned trade-off studies, which does not represent a comparable approach. Some companies mentioned an attempt to apply set-base design with mixed results that led them to give up. It seems that Toyota in Japan has clearly taken a major lead with the approach as a standard practice.
Category 6: Virtual versus physical test
Simulation may significantly reduce the need for physical tests. The level of maturity in replacing physical with virtual models, however, depends at least in part on the level of confidence in virtual results. The pay-off will go straight to the bottom line in terms of time saved and costs reduced.
When comparing practices and maturity levels across companies on the trade-offs of physical versus virtual tests, numerous points have to be clarified. Based on a company’s specific criteria, the maturity or relevance of virtual tests may differ significantly. For some in the aerospace industry, there is no alternative to simulation because there is no prototype to physically test. On the other hand, some tests do not have appropriate simulation models available because of the lack of understanding of the underlying physics, which mandates physical tests.
The objectives of simulation in the automotive area target the reduction of physical tests on the whole vehicle, especially in the early phase of design decisions. The last round of physical test, however, cannot be eliminated given regulatory requirements.
While the reduction of physical tests represents a clear objective for all OEMs and Tier One vendors, the results are mixed. Some with the OEMs mention a reduction of one round of tests with one less prototype series. Others report only minor benefit from simulation on reducing the need for physical tests. The objective of simulation in aerospace targets the best match for the product with customer requirements with the highest reliability at the lowest cost of manufacturing and operation. No surprise givens its long involvement in the technology, aerospace comes in first in terms of maturity and confidence in simulation. The level of confidence in simulation is very high in the aerospace industry, where most of the FEA solutions on the market today were first introduced. That contrasts with automotive, where simulation is trusted in general for design decision support, but not for final validation.
After lagging in applying the approach for many years, the consumer product sector is moving very rapidly into virtual testing, from early concept design to final testing and the simulation of the consumer experience.
At the time of this writing in 2007, Michel Vrinat was PLM Research Director at Collaborative Product Development Associates, LLC, which was acquired in 2011 by CIMdata.